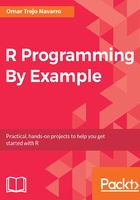
Creating a new dataset with what we've learned
What we have learned so far in this chapter is that age, education, and ethnicity are important factors in understanding the way people voted in the Brexit Referendum. Younger people with higher education levels are related with votes in favor of remaining in the EU. Older white people are related with votes in favor of leaving the EU. We can now use this knowledge to make a more succinct data set that incorporates this knowledge. First we add relevant variables, and then we remove non-relevant variables.
Our new relevant variables are two groups of age (adults below and above 45), two groups of ethnicity (whites and non-whites), and two groups of education (high and low education levels):
data$Age_18to44 <- ( data$Age_18to19 + data$Age_20to24 + data$Age_25to29 + data$Age_30to44 ) data$Age_45plus <- ( data$Age_45to59 + data$Age_60to64 + data$Age_65to74 + data$Age_75to84 + data$Age_85to89 + data$Age_90plus ) data$NonWhite <- ( data$Black + data$Asian + data$Indian + data$Pakistani ) data$HighEducationLevel <- data$L4Quals_plus data$LowEducationLevel <- data$NoQuals
Now we remove the old variables that were used to create our newly added variables. To do so without having to manually specify a full list by leveraging the fact that all of them contain the word "Age", we create the age_variables logical vector, which contains a TRUE value for those variables that contain the word "Age" inside (FALSE otherwise), and make sure we keep our newly created Age_18to44 and Age_45plus variables. We remove the other ethnicity and education levels manually:
column_names <- colnames(data) new_variables <- !logical(length(column_names)) new_variables <- setNames(new_variables, column_names) age_variables <- sapply(column_names, function(x) grepl("Age", x)) new_variables[age_variables] <- FALSE new_variables[["AdultMeanAge"]] <- TRUE new_variables[["Age_18to44"]] <- TRUE new_variables[["Age_45plus"]] <- TRUE new_variables[["Black"]] <- FALSE new_variables[["Asian"]] <- FALSE new_variables[["Indian"]] <- FALSE new_variables[["Pakistani"]] <- FALSE new_variables[["NoQuals"]] <- FALSE new_variables[["L4Quals_plus"]] <- FALSE new_variables[["OwnedOutright"]] <- FALSE new_variables[["MultiDeprived"]] <- FALSE
We save our created data_adjusted object by selecting the new columns, create our new numerical variables for the new data structure, and save it as a CSV file:
data_adjusted <- data[, new_variables] numerical_variables_adjusted <- sapply(data_adjusted, is.numeric) write.csv(data_adjusted, file = "data_brexit_referendum_adjusted.csv")