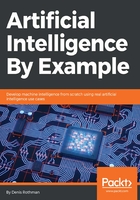
Summary
This chapter led artificial intelligence exploration one more step away from neuroscience to reproduce human thinking. Solving a problem like a machine means using a chain of mathematical functions and properties.
The further you get in machine learning and deep learning, the more you will find mathematical functions that solve the core problems. Contrary to the astounding amount of hype, mathematics relying on CPUs is replacing humans, not some form of alien intelligence.
The power of machine learning with beyond-human mathematical reasoning is that generalization to other fields is easier. A mathematical model, contrary to the complexity of humans entangled in emotions, makes it easier to deploy the same model in many fields. The models of the first three chapters can be used for self-driving vehicles, drones, robots in a warehouse, scheduling priorities, and much more. Try to imagine as many fields you can apply these to as possible.
Evaluation and measurement are at the core of machine learning and deep learning. The key factor is constantly monitoring convergence between the results the system produces and the goal it must attain. This opens the door to the constant adaptation of the weights of the network to reach its objectives.
Machine evaluation for convergence through a chess example that has nothing to do with human thinking proves the limits of human intelligence. The decision tree example can beat most humans in classification situations where large amounts of data are involved.
Human intelligence is not being reproduced in many cases and has often been surpassed. In those cases, human intelligence just proves that intelligence can solve a problem, nothing more.
The next chapter goes a step further from human reasoning with self-weighting neural networks and introduces deep learning.